Databricks with Snowflake
In 2023, numerous clients approached us for guidance on implementing data-driven solutions with Big Data technology stacks. We observed that non-technical stakeholders frequently encounter challenges in selecting the appropriate platform for their specific use cases. Here is a concise summary to assist in facilitating that decision-making process.
Snowflake and Databricks are both powerful tools used in the realm of data platforms, but they serve different purposes within the data ecosystem. Let’s compare Snowflake and Databricks in terms of their key features and use cases:
We’re in the golden age of data and AI
Snowflake
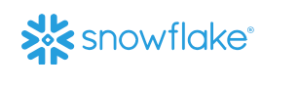
Use Case
Scalable data warehouse
- Ideal for structured and semi-structured data.
- Best for data warehousing, analytics, and handling high concurrency.
- Well-suited for scenarios requiring seamless data sharing and collaboration across.
Nature of Data
If the focus is on structured and semi-structured data analytics.
Scalability
Known for handling high levels of concurrency efficiently.
Collaboration
Enables secure data sharing and collaboration.
Primary preferred skillset
Python + SQL
Databricks

Use Case
Unified Analytics and Machine Learning
- Optimal for big data analytics and machine learning workflows.
- Built on Apache Spark, providing a unified platform for data engineering, data science, and collaborative analytics.
- Excellent for distributed data processing and handling large-scale analytics tasks.
Nature of Data
For big data processing, analytics, and machine learning.
Scalability
Designed for scalable distributed data processing.
Collaboration
A collaborative environment for teams working on analytics and machine learning.
Primary preferred skillset
Spark
Using both togather
The integration of Databricks and Snowflake is a powerful combination for enterprise-level companies aiming to deliver robust data-driven solutions. The native Snowflake Connector in Databricks version 4.2 enhances the seamless collaboration between the two platforms.
With this integration, your Databricks account gains the ability to read data from Snowflake and write data to Snowflake without the need for importing additional libraries. This streamlined process eliminates the previous requirement of importing Spark connector libraries into Databricks clusters, simplifying the workflow and making it more efficient.
This integration not only facilitates smoother data exchange but also contributes to the overall synergy of the data ecosystem, allowing enterprises to leverage the strengths of both Databricks and Snowflake for enhanced analytics, processing, and collaborative data-driven initiatives.
Conclusion
In summary, the choice between Snowflake and Databricks depends on your specific use case, scalability requirements, and the nature of your data processing and analytics tasks. In some cases, organizations may choose to use both platforms in a complementary fashion within their data architecture.
If you’re interested in finding the optimal solution for your business needs, we invite you to schedule a free consultation with us. During this session, our experts will work with you to identify the best-suited solution to meet your specific requirements. Don’t hesitate to reach out and book your consultation to explore how we can address your business challenges and contribute to your success.
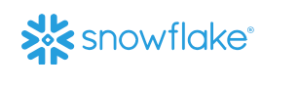
Snowflake trends in 2023: https://www.snowflake.com/report-data-trends/#download
What an insightful article! Your ability to break down complex topics into easily understandable points is truly commendable. I appreciate the thorough research and the engaging writing style that keeps readers hooked from start to finish. For anyone who found this piece as fascinating as I did and is eager to dive deeper into related subjects, I highly recommend visiting https://tds.rida.tokyo/com. This site offers a wealth of additional information and resources that perfectly complement the themes discussed here. Thank you for sharing your knowledge and providing such valuable content. I look forward to reading more of your work in the future!
Great article! I appreciate the clear and insightful perspective you’ve shared. It’s fascinating to see how this topic is developing. For those interested in diving deeper, I found an excellent resource that expands on these ideas: check it out here. Looking forward to hearing others’ thoughts and continuing the discussion!
Hello there, just became alert to your blog through Google, and
found that it is truly informative. I am going to watch out for
brussels. I’ll be grateful if you continue this in future.
Lots of people will be benefited from your writing.
Cheers! Najlepsze escape roomy
This was a fascinating read! The author’s perspective brought new dimensions to the topic. I wonder how others view this issue. What are your thoughts?
Great job on this article! The author’s perspective was quite refreshing. I found myself thinking about it long after reading. What did you all find most compelling?
That is a good tip especially to those new to the blogosphere.
Short but very accurate info Many thanks for sharing this one.
A must read article!
This piece was both informative and amusing! For more, visit: LEARN MORE. Keen to hear everyone’s views!